Empirical Evaluation of Adaptive Optimization on the Generalization Performance of Convolutional Neural Networks
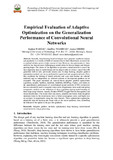
View/ Open
Date
2021-10Author
Wanjau, Stephen
Wambugu, Geoffrey
Oirere, Aaron
Metadata
Show full item recordAbstract
Recently, deep learning based techniques have garnered significant interest
and popularity in a variety of fields of research due to their effectiveness in search for
an optimal solution given a finite amount of data. However, the optimization of these
networks has become more challenging as neural networks become deeper and datasets
growing larger. The choice of the algorithm to optimize a neural network is one of the
most important steps in model design and training in order to obtain a model that will
generalize well on new, previously unseen data. In deep learning, adaptive gradient
optimization methods are mostly preferred for supervised and unsupervised tasks. First,
they accelerate the training of neural networks and since mini batches are selected
randomly and are independent, an unbiased estimate of the expected gradient can be
computed. This paper examined six state-of-the-art adaptive gradient optimization
algorithms, namely, AdaMax, AdaGrad, AdaDelta, RMSProp, Nadam, and Adam on
the generalization performance of convolutional neural networks (CNN) architecture
that are extensively used in computer vision tasks. Experiments were conducted giving
comparative analysis on the behaviour of these algorithms during model training on
three large image datasets, namely, Fashion-MNIST, Kaggle Flowers Recognition and
Scene classification. The results show that Adam, Adadelta and Nadam finds the global
minimum faster in the experiments, have a better convergence curve, and higher test
set accuracy in experiments using the three datasets. These optimization approaches
adaptively tune the learning rate based only on the recent gradients; thus, controlling
the reliance of the update on the past few gradients.